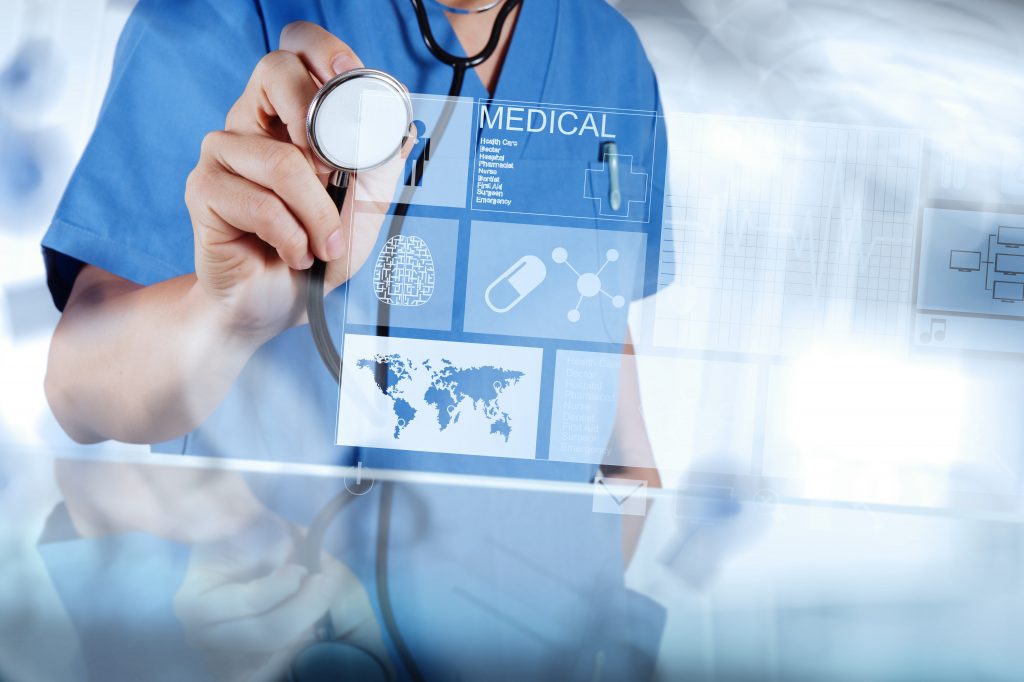
Journal of Advanced Research 37 (2022) 1–18
Cross-tissue single-cell transcriptomic landscape reveals the key cell subtypes and their potential roles in the nutrient absorption and metabolism in dairy cattle
Jia-Jin Wu a,b,1, Senlin Zhu a,b,1, Fengfei Gu a,b, Teresa G. Valencak a, Jian-Xin Liu a,b,c, Hui-Zeng Sun a,b,c,⇑
a Institute of Dairy Science, College of Animal Sciences, Zhejiang University, Hangzhou 310058, China
b Ministry of Education Key Laboratory of Molecular Animal Nutrition, Zhejiang University, Hangzhou 310058, China
c Ministry of Education Innovation Team of Development and Function of Animal Digestive System, Zhejiang University, Hangzhou 310058, China
h i g h l i g h t s
●Discover 55 cell types and their specific markers in the first single-cell atlas of cattle;
●Identify and verify 3 epithelial progenitor-like cell subtypes in the forestomach
●Reveal vital but nonimmune functions of neutrophils in the mammary gland;
●Uncover key cell subtypes with preferential nutrient uptake;
●Find Th17 cells regulate epithelial cells responding to nutrient transport in the forestomach.
g r a p h i c a l a b s t r a c t
a r t i c l e
i n f o
a b s t r a c t
Article history:
Received 1 September 2021
Revised 2 November 2021
Accepted 19 November 2021
Available online 24 November 2021
Keywords:
Dairy cattle
Intercellular communication Metabolic features
Nutrient absorption Single-cell landscape
Introduction: Dairy cattle are a vitally important ruminant in meeting the demands for high-quality ani- mal protein production worldwide. The complicated biological process of converting human indigestible biomass into highly digestible and nutritious milk is orchestrated by various tissues. However, poorly understanding of the cellular composition and function of the key metabolic tissues hinders the improve- ment of health and performance of domestic ruminants.
Objectives: The cellular heterogeneity, metabolic features, interactions across ten tissue types of lactating dairy cattle were studied at single-cell resolution in the current study.
Methods: Unbiased single-cell RNA-sequencing and analysis were performed on the rumen, reticulum, omasum, abomasum, ileum, rectum, liver, salivary gland, mammary gland, and peripheral blood of lac- tating dairy cattle. Immunofluorescences and fluorescence in situ hybridization were performed to verify cell identity.
Results: In this study, we constructed a single-cell landscape covering 88,013 high-quality (500 < genes < 4,000, UMI < 50, 000, and mitochondrial gene ratio < 40% or 15%) single cells and identified
Peer review under responsibility of Cairo University.
* Corresponding author at: Institute of Dairy Science, College of Animal Sciences, Zhejiang University, Hangzhou 310058, China.
E-mail address: [email protected] (H.-Z. Sun).
1 These authors contributed equally to this work.
https://doi.org/10.1016/j.jare.2021.11.009
2090-1232/© 2022 The Authors. Published by Elsevier B.V. on behalf of Cairo University.
This is an open access article under the CC BY-NC-ND license (http://creativecommons.org/licenses/by-nc-nd/4.0/).
55 major cell types in lactating dairy cattle. Our systematic survey of the gene expression profiles and metabolic features of epithelial cells related to nutrient transport revealed cell subtypes that have pref- erential absorption of different nutrients. Importantly, we found that T helper type 17 (Th17) cells (highly expressing CD4 and IL17A) were specifically enriched in the forestomach tissues and predominantly inter- acted with the epithelial cell subtypes with high potential uptake capacities of short-chain fatty acids through IL-17 signaling. Furthermore, the comparison between IL17RAhighIL17RChigh cells (epithelial cells with IL17RA and IL17RC expression levels both greater than 0.25) and other cells explained the impor- tance of Th17 cells in regulating the epithelial cellular transcriptional response to nutrient transport in the forestomach.
Conclusion: The findings enhance our understanding of the cellular biology of ruminants and open new avenues for improved animal production of dairy cattle.
© 2022 The Authors. Published by Elsevier B.V. on behalf of Cairo University. This is an open access article
under the CC BY-NC-ND license (http://creativecommons.org/licenses/by-nc-nd/4.0/).
Introduction
The combination of the increasing global population and poten- tially a reduced agricultural productivity due to climate change represents a major challenge to meeting demand for nutritious foods for humans [1–2]. Ruminants possessing a four-chambered stomach (consisting of the rumen, reticulum, omasum, and aboma- sum) are physically and physiologically different from monogastric animals and can convert indigestible and low-quality plant polysaccharides into absorbable nutrients. Lactating dairy cattle, economically a highly important livestock species, plays an essen- tial role by providing high quality proteins via milk to meet the demand around the world.
A homeostatic orchestration of diverse metabolic tissues is nec- essary for the maintenance of lactation. The gastrointestinal tract is the central site of feed digestion, nutrient uptake, and contributes significantly endocrinologically to the orchestration of metabolism in the ruminant [3]. Short-chain fatty acids (SCFAs) produced in the forestomaches are largely absorbed across the epithelium of the rumen and of the omasum, which ultimately provides up to 70% of daily energy requirements [4]. Salivary buffer secreted by the salivary gland transferring into the rumen also significantly affects the amount of produced SCFAs [5]. SCFAs and other nutrients are extracted into the blood before being transported to the liver for various metabolic processes and finally to the mammary gland [6]. Before the end of the last century, substantial progress was reached in identifying the molecular function of these key tissues. The gastrointestinal tract and other key metabolic tissues (liver, mammary and salivary glands, and peripheral blood) have complex cellular compositions. However, the underlying cell types and composition of tissues, and ultimately functions, are not com- pletely understood. Exploring the cell types/subtypes, their gene expression profiles and interactions may provide a cellular digital reference map for ruminant biology and lead to discover new potential candidate for future increases in animal production. Pre- vious study has reported the roles of bulk RNA sequencing and other omics approaches in the identification of potential metabolic mechanisms across three key metabolic organs (rumen, liver, and mammary gland) in lactating dairy cows [6]. However, to date, there is a lack of integrative profiling of transcriptome and meta- bolic features across multiple metabolic tissues at single-cell resolution.
Recent advances in single-cell RNA sequencing (scRNA-seq) enable the identification of cell subtypes and elucidation of single-cell transcriptomic dynamics [7–9]. Here, we performed scRNA-seq to construct the single-cell atlases of 10 key metabolic tissue types, including the rumen, reticulum, omasum, abomasum, ileum, rectum, liver, salivary gland, mammary gland, and periph- eral blood of lactating dairy cattle. We identified the major cell subsets with signature genes and then created a global landscape of metabolic features of epithelial cell subtypes across tissues. Spe-
cial epithelial cell subtypes that preferentially take up SCFAs or other important nutrients were identified in our study. We revealed that Th17 cells play a vital role in the regulation of the forestomach epithelial transcriptional response for nutrient trans- port. Our study opens potential avenues to further improve the health and milk production in dairy cattle.
Material and methods
Ethics statement
This study was approved by the Animal Care Committee at Zhe- jiang University (approval number ZJU202017326). All applicable institutional and national guidelines for the care and use of ani- mals were followed.
Sample collection
Ten types of metabolic tissues were freshly sampled from six Holstein dairy cows (one to three replicates per tissue type in gen- eral; Fig. S1A) selected from commercial dairy farms under the same management. All animals were fed the same diet (corn- based high grain) with a forage-to-concentrate ratio of 45:55 (dry matter basis). Cows were humanely euthanized after undergo- ing a 12-h fast to collect tissue samples. Clinical information of the animals is presented in Fig. S1A.
Sample preparation for single-cell sequencing
The rumen, reticulum, omasum, abomasum, ileum, and rectum tissues isolated from cattle were stripped of the outer muscle lay- ers and then minced into 10 × 0.5 mm2 pieces on ice with scissors. The tissues were incubated in a 37 °C water bath with 20 mM EDTA for 30 min, rinsed with DPBS and chopped into 1-mm pieces. Tis- sue pieces were transferred to a 15-mL centrifuge tube and resus- pended in 0.25% trypsin-EDTA (Gibco). After incubation in a 37 °C water bath for 5 min, the centrifuge tube containing tissues was inserted into ice for 2 min, and prechilled HBSS was added to stop the digestion. After centrifugation at 300 × g for 2 min at 4 °C, the supernatant was discarded, and the samples were washed twice with cold HBSS and then resuspended in dissociation enzymes. Samples were treated with different dissociation enzymes for dif-
ferent durations, for example, rumen samples were incubated with multiple enzymes (1.5 mg/ml collagenase I, 1.5 mg/ml collagenase
IV, and 1.5 mg/ml dispase, 100 U/ml hyaluronidase, and 50 U/ml DNase I) for 30 min at 37 °C (Fig. S1B and C). In this dissociation step, more details of other tissues dissociated by specific enzymes for different times were available in the Fig. S1B. The digestion was
stopped by adding 10% FBS, followed by a filtration step through 70-lm and 30-lm SmartStrainer (Miltenyi Biotec, Bergisch Glad-
bach, Germany). Samples were centrifuged at 300 × g for 5 min at 4 °C and then resuspended in 2 mL of HBSS. Dissociated cells were centrifuged at 300 × g for 5 min at 4 °C, washed twice with 1 × PBS with 0.04% BSA, centrifuged at 300 × g for 5 min at 4 °C, and resus- pended in 1 × PBS with 0.04% BSA.
For the salivary gland samples, the isolated tissues from the parotid gland were transferred into cold 1 × PBS, blood and fat were removed, and samples were chopped into 0.5-mm pieces. After incubation with dissociation enzymes (Fig. S1B), the samples were passed through 70-lm and 30-lm SmartStrainers (Miltenyi Biotec, Bergisch Gladbach, Germany) and then centrifuged at 300 × g for 5 min at 4 °C. Cells were resuspended in 300 lL of RPMI 1640 medium (Gibco), and then 3 mL of Red Blood Cell Lysis Solu- tion (Miltenyi Biotech, Bergisch Gladbach, Germany) was added for 3 min. The samples were centrifuged at 300 × g for 5 min at 4 °C and resuspended in 2 mL of RPMI 1640 medium. Cells were washed twice with 1 × PBS with 0.04% BSA, centrifuged at
300 × g for 5 min at 4 °C, and resuspended in 1 × PBS with 0.04% BSA.
For the liver samples, the isolated tissues were transferred into cold DPBS, minced into 1-mm pieces, and then incubated with dis-
sociation enzymes (Fig. S1B). The samples were then passed through 70-lm and 30-lm SmartStrainer (Miltenyi Biotec, Ber- gisch Gladbach, Germany), centrifuged at 300 × g for 5 min at 4 °C, and resuspended in 2 mL of cold HBSS. Cells were washed twice with 1 × PBS with 0.04% BSA, centrifuged at 300 × g for 5 min at 4 °C, and resuspended in 1 × PBS with 0.04% BSA.
For the mammary gland samples, the isolated tissues were cut into 5-mm pieces and washed using 1 × PBS (with 5% FBS) after removing the adipose tissue. Subsequently, the samples were incu- bated in a bath with 20 mM EDTA (with 5% FBS) at 100 rpm for 20 min at 37 °C and then incubated with dissociation enzymes
(Fig. S1B). The mixture was passed through 70-lm and 30-lm
SmartStrainers, transferred into a new EP tube, and centrifuged at 300 × g for 5 min at 25 °C. After removing the supernatant, the cells were washed with 3 mL of HBSS with 5% FBS and cen- trifuged at 300 × g for 5 min.
For peripheral blood samples, PBMCs and neutrophils were enriched by Ficoll (HY2015, TBD) separation.
The MACS Dead Cell Removal Kit (Miltenyi Biotec, Bergisch Gladbach, Germany) was used to remove dead cells and cellular debris following the manufacturer’s recommendations. After that, the cells were counted and checked for viability via trypan blue using a Countess II Automated Cell Counter. Finally, all the single-cell suspensions of each sample were free of cell debris
and had high viability (over 85%). The cells were further diluted to a concentration of 700–1200 cells/lL with 1 × PBS with 0.04% BSA for 10X Genomics sequencing.
Single-cell capture, library preparation and sequencing
Sorted live cells were captured for library preparation using Chromium Single Cell 30 Reagent Kits v3 (10X Genomics). The libraries were checked for quality using the Agilent Bioanalyzer High Sensitivity chip, and the libraries were sequenced on the NovaSeq 6000 platform in a 150-bp paired-ended manner.
scRNA-seq computational analysis
Sequencing results were demultiplexed and converted to FASTQ format using Illumina bcl2fastq software. Sample demultiplexing, barcode processing and single-cell 30 gene counting were calcu- lated using CellRanger (version 3.1.0), and scRNA-seq data were aligned to the ARS-USD1.2 cattle reference genome.
The CellRanger outputs were loaded into Seurat [10] (version 4.0.3) for dimensional reduction, clustering, and analysis of
scRNA-seq data. Overall, cells with<500 and more than 4,000 detected genes, UMI counts more than 50,000, and a mitochondrial gene ratio of more than 40% (15% for the peripheral blood dataset) were considered low-quality cells and removed from the dataset. The DoubletFinder [11] package (version 2.0.3) was also used to remove doublets. To visualize the data, we further reduced the dimensionality of all high-quality cells using Seurat (version 4.0.3) and used the t-distributed stochastic neighbor embedding (t-SNE) algorithm to project the cells into two-dimensional space. We performed batch correction using Harmony [12] (version 0.1.0) for data integration between samples. The analysis was per- formed using the R package Seurat (version 4.0.3) with the follow- ing steps: (1) the ‘‘NormalizeData” function was applied to calculate the expression value of genes; (2) the function ‘‘FindVariableGenes” was performed to select variable genes, and the expression levels of these genes were scaled using the ‘‘ScaleData” function; (3) PCA (principal component analysis) anal- ysis was performed in variable gene space using the ‘‘RunPCA” function, and R package Harmony (version 0.1.0) was used to cor- rect batch effects; (4) the ‘‘FindClusters” function with an appropri- ate resolution was used to perform cell clustering; (5) the function ‘‘RunTSNE” was used to visualize cells; (6) the ‘‘FindAllMarkers” function was used to determine the differentially expressed genes (DEGs) or marker genes (|‘avg_logFC’| greater than 0.25 and ‘p_- val_adj’ < 0.05); (7) the AUC value for the marker genes was also calculated using the function ‘‘FindAllMarkers”. An AUC value of 1 means that the gene is a perfect classifier for a given cluster, and an AUC value of 0.5 implies that the gene has no predictive value for cluster identity. The cell cluster identity was assigned by manual annotation based on the expression and AUC value of known marker genes (Tables S1 and S2).
To further understand the composition of cell in each tissue type, we processed the scRNA-seq data for all ten tissue types (ru- men, reticulum, omasum, abomasum, ileum, rectum, salivary gland, liver, mammary gland, and peripheral blood) separately fol- lowing the same analysis pipeline to generate a single-cell atlas for each tissue type. To more clearly show the workflow of single-cell RNA-seq analysis, we have added the short schematic diagrams in the Fig. S2.
Differential expression analysis
Epithelial cells with IL17RA and IL17RC expression levels both higher than 0.25 were separated into IL17RAhighIL17RChigh cells and others were separated into other cells (control group) in the rumen, reticulum, and omasum. Differential gene expression anal- ysis of epithelial cells between the IL17RAhighIL17RChigh and control groups was performed using the Wilcoxon rank-sum test as imple- mented in the ‘‘FindMarkers” function from the Seurat package (version 4.0.3). Only genes expressed in more than 15% of the cells in groups were considered. DEGs were identified to generate upregulated DEG datasets (LogFC greater than 0.25, adjusted p value < 0.05) for the IL17RAhighL17RChigh group.
Cell cycle analysis
Cell cycle stage annotation of each cell among the MC_1 and MC_2 of rumen tissue was performed using the ‘‘CellCycleScoring” function in Seurat (version 4.0.3), which assigns each cell a score based on the expression of marker genes for G2/M phase and marker genes for S phase.
Enrichment analysis
GO term enrichment analysis was performed using the function ‘‘enrichGO” in the clusterProfiler R package [13] (version 4.0.5)
based on the dataset ‘org.Bt.eg.db’. The DEGs were mapped to the ‘‘bta” KEGG pathway database using the ‘‘enrichKEGG” function of the clusterProfiler R package (version 4.0.5). Gene set enrich- ment analysis (GSEA) was performed using the ‘‘GSEA” function in the clusterProfiler R package (version 4.0.5) to identify gene sets that are enriched in cell types, and the results were visualized with
the enrichplot R package (version 1.12.3) (https://github.com/ YuLab-SMU/enrichplot).
Pseudotime analysis
To model differentiation trajectories, we performed trajectory analysis using Monocle3 [14] (version 1.0.0) for all neutrophils in peripheral blood and mammary glands, according to the general
pipeline (https://cole-trapnell-lab.github.io/monocle3/).
Metabolic heterogeneity analysis
We performed a computational pipeline according to published methods [15] (https://github.com/LocasaleLab/Single-Cell-Meta-
bolic-Landscape) with some modifications to study the metabolic heterogeneity of the epithelial cell types across tissues of dairy cat- tle at single-cell resolution. In brief, the digital gene expression matrices with raw counts of epithelial cell types that were selected from each tissue were merged together. The merged gene expres- sion matrix and the gene lengths were used as the input. The gene expression levels between cell types were calculated using the deconvolution normalization method. The pathway activity was calculated with default parameters, and the results were visualized by the pheatmap R package (version 1.0.12).
Ligand-receptor interaction analysis
To investigate cell–cell communications from scRNA-seq data, we identified the inferred cellular communication patterns using the CellChat [16] R package (version 0.5.0) with default parame- ters. We grouped clusters within Th17 subtypes because of their low degree of heterogeneity based on their expression profiles in the reticulum or omasum dataset.
Gene set scoring analysis
Genes within the gene sets ‘‘Monocarboxylic acid transport” and ‘‘Saliva secretion” are listed in Table S3. The ‘‘AddModuleS- core” function of Seurat R package (version 4.0.3) was used to com- pute the signature score of the gene set in each cell type. The differences in the signature scores across cell types were evaluated by a two-sided Wilcoxon rank sum test. Mean values labeled with- out a common letter were defined as significantly different (the order of the letters (from ‘‘a” to ‘‘g”) was sorted according to mean values from high to low, p. adjusted value < 0.05).
Widely targeted metabolomics analysis
The epithelial tissues of rumen, reticulum, omasum, abomasum, ileum, and rectum were homogenized with 1,000 ul of ice-cold methanol/water (70%, v/v) and cold steel balls for 3 min at
30 Hz. After being whirled for 1 min without steel balls and 15 min standing, samples were centrifuged at 4℃, 12,000 rpm for
10 min. The supernatant was collected for LC-MS/MS analysis. QTRAP® LC-MS/MS System equipped with an ESI Turbo Ion-Spray interface is operated in positive and negative ion mode and con- trolled by Analyst software (version 1.6.3). The ESI source opera- tion parameters were as follows: source temperature 500℃; ion spray voltage 5500 V (positive), —4500 V (negative); ion source
gas I, gas II, curtain gas were set at 50, 50, and 25 psi, respectively; the collision gas was high. Instrument tuning was performed with 10 lmol/l polypropylene glycol solutions in QQQ modes. Instru-
ment mass calibration was performed with 100 lmol/l polypropy-
lene glycol solutions in LIT modes. A specific set of MRM transitions were monitored for each period according to the metabolites eluted within this period. The mass spectrometric data was analyzed using the Analyst software (version 1.6.3), and in a qualitative analysis of the metabolites based on the retention time of the detected substance and secondary spectral data against the metware database.
Immunofluorescence
Multiplex immunostaining was performed on 5-lm-thick, formalin-fixed, paraffin-embedded rumen, reticulum, and omasum tissue slides. Briefly, antigen retrieval was accomplished by microwaving the sections at 98℃ in 10 mM Tris-EDTA (pH 8.0). Slides were blocked with 3% methanol-hydrogen peroxide solution at room temperature for 25 min and then washed three times (5 min each time) with PBS (pH 7.4). After blocking with 0.5%
BSA for 30 min, the primary antibody was added to the slides for incubation at 4 °C overnight. After washing with PBS (pH 7.4), the slides were incubated with polymer horseradish peroxidase (HRP)-conjugated antibody specific to rabbits. After rinsing, CY3-
TSA or FITC-TSA was added to each slide and incubated at room temperature. Antigen retrieval was performed on stained slides to prepare them for staining to detect the next target protein. Nuclei were counterstained with DAPI. The primary antibodies used were anti-KRT14 (ET1610-42, HUABIO), anti-MKI67 (ER1706-46, HUABIO), anti-KRT6A (ET1611-70, HUABIO), and anti-GJA1 (ER1802-88, HUABIO).
Fluorescence in situ hybridization (FISH)
FISH was performed on formalin-fixed, paraffin-embedded tis- sue slides. In brief, after dewaxing, dehydration, and antigen recov- ery, proteinase K (20 mg/ml) working solution was added to slices
and incubated at 37 °C for 10 min. After blocking endogenous per-
oxidase with 3% methanol-hydrogen peroxide solution for 15 min at room temperature, slides were incubated for 1 h at 37 °C with prehybridization solution (Servicebio, Wuhan, China). The CD4 probe hybridization solution was added to the slices for incubation in a humidity chamber overnight at 37 °C. After blocking with rabbit serum for 30 min at room temperature, the slides were incu-
bated with anti-DIG-HRP for 50 min at 37 °C. FITC-TSA or CY3-TSA was added to slides and incubated at room temperature for 5 min. After rinsing, the stained slides were prepared for staining to detect the next target mRNA, IL17A. The RNA probes specific to bovines used for this study were CD4 (5‘-DIG-GAGGGACTCTC CAAAGTCAAGGTCAGGC-DIG-3') and IL17A (5‘-DIG-GGAAGTTCTT GTCCTCAGTAGGTGGGCAGC-DIG-3').
Statistical analysis
For data of metabolites, the differences among groups were per- formed using ordinary one-way ANOVA test and the correct for multiple comparisons was using the Tukey method in GraphPad Prism software (version 8.0). The data in figure are presented as the means ± SD.
Data availability
The raw and processed sequencing data of the reticulum, oma- sum, abomasum, ileum, rectum, salivary gland, and liver, mam- mary gland, and peripheral blood as well as the processed data
of the rumen generated in this study have been submitted to the NCBI Gene Expression Omnibus (GEO; https://www.ncbi.nlm.nih.- gov/geo/query/acc.cgi?acc = GSE176512) under accession number GSE176512, and the secure token ‘‘ejcfqqqghvopbkl” allow review of record GSE176512. The raw data of the rumen were collected from our previously published data in the SRA database under accession number SRP321626 (https://www.ncbi.nlm.nih.gov/sra/
?term = SRP321626). This study did not generate any unique code. All software is freely or commercially available.
Results
Construction of single-cell atlases by scRNA-seq in dairy cattle
To understand the cell type composition and transcriptional profiles of various tissues that are vital for the lactation process in dairy cattle, we generated a single-cell atlas for lactating Hol- stein cattle covering 10 different tissue types, including the rumen, reticulum, omasum, abomasum, ileum, rectum, liver, salivary gland, mammary gland, and peripheral blood. We totally obtained more than 4,622 million sequencing reads, and on average, more than 385 million sequencing reads for each tissue sample (Fig. S3A). After quality control, a total of 88,013 high-quality sin- gle cells were obtained for downstream analyses. These included 25,828 rumen, 6,257 reticulum, 10,097 omasum, 3,908 abomasum, 9,194 ileum, 5,600 rectum, 7,370 liver, 2,213 salivary gland, 11,436 mammary gland, and 6,110 peripheral blood cells (Fig. S3B). We combined all 88,013 cells in the cluster analysis across ten tissues with batch effect correction. We identified 90 clusters that could be divided into 55 major cell types (Fig. 1A) based on the expression levels of canonical cell-type-specific markers (Table S1). The dot plot showed that the representative highly expressed marker genes (area under the curve (AUC) ≥ 0.75) could successfully clas- sify cells into these major cell types (Fig. 1B; Table S2).
For each immune cell type, we found that cells from different
tissues clustered together (Fig. 1C and D), which is consistent with the understanding that circulating immune cells, derived from the same lineage, are widely distributed within the mammalian body [17]. Moreover, multiple subtypes were identified for several immune cell types, such as T cells (highly expressing CD3E and CD3D), natural killer T cells (highly expressing CD3E, CTSW, and NKG7), and macrophages (highly expressing CD68), reflecting their largely heterogeneous transcriptional profiles. The epithelial cells displayed tissue distributions, except for subtypes of keratinocytes (rumen, reticulum, and omasum) and intestinal epithelial cell sub- types (ileum and rectum), reflecting the heterogeneity of epithelial cells at both the intra- and inter-tissue levels (Fig. 1C and D).
Cellular heterogeneity in dairy cattle tissues
To further systematically investigate single-cell transcriptomic heterogeneity in individual tissues, we performed t-distributed stochastic neighbor embedding (t-SNE) and differential gene expression analysis separately for each tissue type. Previously unrecognized cell heterogeneity and novel cell subtypes across a wide range of bovine tissues were discovered. After analyzing the forestomach tissues, we defined 23, 17, and 18 clusters with highly expressed genes in the rumen (Fig. 2A; Fig. S4; Table S4), reticulum (Fig. 2B and C; Fig. S5A; Table S5), and omasum tissues (Fig. 2D and E; Fig. S5B; Table S6), respectively. These three tissues have anatomically similarly stratified squamous epithelium [18] con- sisting of keratinocytes that include basal cells (BC), spinous cells (SC), and granule cells (GC). Multiple cell subtypes among BC (highly expressing KRT14) [19–20], SC (highly expressing KRT10, KRT6A, and S100A8) [20–21], and GC (highly expressing DLK2)
[22] were identified in each tissue. We noted that the cluster 6 spi- nous cell subtype in the reticulum and cluster 15 spinous cell sub- type in the omasum shared common gene expression signatures with cluster 10, cluster 14, and cluster 19 spinous cell subtypes of the rumen, which specifically highly expressed GJA1 (Fig. 2C and E; Fig. S4A). GJA1 encodes a gap junction protein that is respon- sible for the transport of nutrients [23]. Immunofluorescence assays for KRT6A and GJA1 further confirmed the presence of channel-gap-like spinous cells (cg-like SC) in the reticulum and omasum tissues (Fig. 2F). Death and renewal of cells occur continuously in the rumen epithelium; thus, it is believed that stem cells/progenitors exist in the stratum basale. However, these cell populations remain largely unknown [24]. Herein, we discov- ered epithelial progenitor-like subtypes (mitotic cells, MC) in the rumen based on the expression of the marker genes KRT14 and MKI67 [21,24] (Fig. S4A), which were further verified by immunofluorescence staining (Fig. 2G). MCs were also found in the reticulum and omasum tissues. There were two MC subtypes named MC_1 and MC_2 in the rumen, in which MC_1 was highly expressed in cell division-related genes, while DNA replication- related genes were enriched in MC_2 (Fig. S4E). This indicates that the major difference between these two MCs was the cell cycle sta- tus, which was also confirmed by cell cycle analysis (Fig. S4F). We observed that almost every epithelial cell type in the rumen, retic- ulum and omasum could be clearly distinguished by its combina- torial set of transcription factors. We observed that the MCs of these three tissues all highly expressed the transcription factors HMGB2, CENPS, and CTCF (Fig. S6).
We also discovered a diversity of cell types in the other tissues of cattle that have not previously been characterized. We identified 16, 26, 16, 21, and 15 cell subtypes in the abomasum (Fig. 3A and B; Table S7), ileum (Fig. 3C and D; Table S8), rectum (Fig. S7A and B; Table S9), liver (Fig. S7C and D; Table S10), and salivary gland (Fig. S7E and F; Table S11) based on the expression of marker genes, respectively. We discovered new cell subtypes in these tis- sues, for example, HES1+ progenitors (high HES1 expression) in the abomasum (Fig. 3B), CCK+ enterocytes (high FABP1 and CCK expression) and GUCA2A+ (high FABP2, GUCA2A and GUCA2B expression) enterocytes in the ileum and rectum of cattle (Fig. 3D; Fig. S7B). Neutrophil cells can migrate out of the circula- tion and infiltrate many healthy tissues in which they acquire tran- scriptional profiles that are unique to the tissue of residence [25]. In this study, we focused on neutrophils (high expression of TGM3 and CXCR1) in peripheral blood and mammary gland tissues (Fig. S8; Tables S12 and S13) and identified their changes in func- tions such as blood vessel development, epithelial tube formation, and response to steroid hormones during migration from periph- eral blood to the mammary gland by using pseudotime analysis (Fig. 3E and F). Our new finding indicates that neutrophils fulfill vital, nonimmune system-related tasks in the mammary gland.
Metabolic landscape of epithelial cell types in different tissues
As metabolism fuels physiological functions and controls cellu- lar behavior, we aimed to assess the enrichment of metabolic path- ways in each epithelial cell subtype in different tissues (excluding proliferative mitotic cells and stem/progenitor cells in the gastroin- testinal tract). In general, the metabolic pathways that were signif- icantly upregulated in the epithelial cell subtype were grouped into 10 categories based on KEGG classifications that reflect differ- ent aspects of cellular metabolism, such as amino acid metabolism, carbohydrate metabolism, and lipid metabolism (Fig. 4A). We observed that the basal and spinous cell subtypes had most of the upregulated metabolic pathways, whereas most of the granule cell subtypes had no significantly upregulated metabolic pathways compared to other cell types in the forestomach tissues (Fig. 4B). In
A C
88,013 cells
tSNE_1
NKT: Natural killer T cell; BC: Basal cell; SC: Spinous cell; GC: Granule cell; Mon: Monocyte; LEC: Lymphatic endothelial cell;PlaC: Plasma cell; Neu:Neutrophil; PanC: Paneth cell; Mac: Macrophage; MC: Mitotic cell; NecC: Neck cell; ChiC: Chief cell; GobC: Goblet cell; MasC: Mast cell; Fib: Fibroblast; Ent: Enterocyte; PitC:
Pit cell; BVEC: Blood vascular endothelial cell; LumC: Luminal cell; AciC: Acinar cell; NK: Natural killer cell; DucC: Duct cell; Myo: Myofibroblast; Hep: Hepatocyte; KupC: Kupffer cell; Cho: Cholangiocyte; TA:
Transit-amplifying cell; SchC: Schwann cell; DC: Dendritic cell
B
VCAN FCN1 S100A9 TGM3 CXCR1 CD68 CD14 CD163 CD5L IRF8 CST3 CD83 CPA3 KIT NKG2A NCR1 KLRB1 CTSW NKG7 CCL5 PRF1 KLRJ1 GNLY CD3D CD3E CD8A CD8B CD4 CD79A CD79B MS4A1 CD19 MZB1 JCHAIN KRT14 KRT5 S100A8 KRT6A DLK2 CELA1 SPDEF TMED6 PGA5 GKN2 GKN1 TFF1 AGR2
ENSBTAG00000050212
SPINK4 TFF3 CD24 DEFB FABP1 FABP2 VIL1 MKI67 STMN1 TOP2A FGB ALB EPCAM
ONECUT1
CA6 BPIFA2B BPIFA2A KRT19 KRT7 KRT18 KRT8 LALBA GLYCAM1 CSN2 CSN1S1 CSN3 LYVE1 PECAM1 VWF
DCN COL1A2 COL3A1 MYH11 MYL9 ACTA2 NCAM1 S100A4 SOX10 PMP22 MAL
Rumen Reticulum
Ileum Rectum Mammary gland
D
tSNE_1
Omasum
Liver Blood
Abomasum Salivary gland
SchC Myo Fib
BVEC_2 BVEC_1 LEC
LumC_3
LumC_2 LumC_1 DucC/LumC DucC
AciC Cho Hep TA
Ent_4 Ent_3 Ent_2 Ent_1 PanC GobC_2
GobC_1 PitC/GobC PitC
ChiC NecC GC SC_2 SC_1 BC_3 BC_2 BC_1 MC
PlaC B
MKI67+ T_2 MKI67+T_1
T_4 T_3 T_2 T_1 NKT_4 NKT_3 NKT_2
NKT_1 NK
MasC DC_2 DC_1
KupC Mac_2 Mac_1 Neu Neu/Mon Mon
Cell types
Average Expression Percent Expressed (%)
Tissue proportions (%)
Rumen Reticulum Omasum Abomasum Ileum
Rectum Liver
Salivary gland Mammary gland Blood
Fig. 1. Construction of the single-cell landscape of the key metabolic tissues in dairy cattle. (A) t-SNE analysis of 88,013 single cells from dairy cattle, with 55 major cell types labeled in different colors. (B) Dot plots showing the expression of representative marker genes with an AUC cutoff ≥ 0.75 for each cell type. (C) t-SNE analysis of 88,013 single cells from dairy cattle, with 10 tissue types labeled in different colors. (D) Proportions of the 55 major cell types in the tissues. AUC: area under the curve.
A Rumen
2
11
4
1
3
0
7
20 5
16
9 8 6 17
18
15 13 14
10 21
0Th17
1GC_1
2GC_2
3GC_3
4GC_4
5SC_1
6SC_2
7SC_3
8BC_1
9γδ T
10cg-like SC_1
11GC_5
12MC_1
13BC_2
14cg-like SC_2
15MC_2
16GC_6
17SC_4
18CD4- CD8- T
B Reticulum
16
5 7
11 0
8
2
3 15 4
6 10
12
9 1
C
0GC_1
1Th17_1
2SC_1
3BC_1
4γδ T
5GC_2
6cg-like SC
7GC_3
8BC_2
9MC
10BC_3
11cDC2
12SC_2
13Th17_2
14MKI67+ Th17
15CD4- CD8- T
16VSMC
VSMC: Vascular smooth
KRT14 MKI67 TOP2A
MT1E 2
PLAC8 TUBA1B 1
GSTO1 0
TM4SF1 −1
S100A9
PHGR1 −2
MDH1 S100A8 KRT6A KRT10 CLDN4 GJA1 IGFBP5 BREH1 LYPD3 PDK4 CCN1 THBS1 CFB SERTAD4 SYNE2
12 22
19
D Omasum
19
cg-like SC_3
20MKI67+ Th17
21cDC2
22BVEC
cDC2: conventional type 2 dendritic cell
muscle cell
13
14
E
GRIA3 LAMB1 CA3 CDKN1C DLK2
Reticulum epithelial cell clusters
KRT14
17 12
14 3
6
4
13
5 9
15
2
8
10 0
7 1
16
11
F
0 Th17_1
1 Th17_2
2SC_1
3GC_1
4GC_2
5SC_2
6GC_3
7MC
8BC_1
9Th17_3
10BC_2
11γδ T
12cDC2
13GC_4
14BVEC
15cg-like SC
16MKI67+ Th17
17LEC
Omasum epithelial cell clusters
G
MKI67 TOP2A MT1E MT2A
FABP5 0
UHRF1
PCNA −1
DTYMK
GSTO1 −2
NET1 ADAMTSL5 IDH1 KRT19 GJA1 GJB2 WFDC18 BPIFA2C S100A8 KRT6A CLDN4 KRT10 CDKN1C SPRY1 AOX1
IER3 RCAN1 AHCY KRT42 BTBD7 ANGPTL4 EPN2 LCAT DLK2
KRT6A
GJA1
Merge
KRT6A
GJA1
Merge
Reticulum Omasum
KRT14
MKI67
Merge
Fig. 2. Construction of single-cell landscapes of the forestomach tissues. (A, B, D) t-SNE maps of the rumen (A), reticulum (B), and omasum (D) single-cell data. Cells are colored by cell types. (C, E) Heatmap showing the representative highly expressed marker genes for epithelial cell subtypes in the reticulum (C) and omasum (E). Color scale: red, high expression; blue, low expression. (F) Coimmunostaining of KRT6A with GJA1 in reticulum and omasum tissues. Scale bars, 20 lm. (G) Coimmunostaining of KRT14 and MKI67 in rumen tissues. Scale bars, 20 lm. BC: basal cell; BVEC: blood vascular endothelial cell; cg-like SC: channel-gap-like spinous cell; GC: granule cell; LEC: lymphatic endothelial cell; MC: mitotic cell; SC: spinous cell. (For interpretation of the references to color in this figure legend, the reader is referred to the web version of this article.)
Fig. 3. Cellular heterogeneities in the abomasum, ileum, mammary gland, and peripheral blood. (A, C) The t-SNE maps of the abomasum (A) and ileum (C) single-cell data. Cells are colored by cell types. (B, D) Dot plots showing the expression of representative marker genes for each cell type in the abomasum (B) and ileum (D). (E) Pseudotime trajectory analysis corresponding to the differentiation of neutrophils migrating from peripheral blood to the mammary gland. Cells are colored by pseudotime and tissue types. (F) Gene modules that change as cells progress along the trajectory. The colors from blue to red indicate low to high aggregate module scores. Representative cell type-specific GO terms are listed on the right. BVEC: blood vascular endothelial cell; cDC1: conventional type 1 dendritic cell; LEC: lymphatic endothelial cell; NKT: natural killer T cell; TA: transit-amplifying cell; VSMC: vascular smooth muscle cell. (For interpretation of the references to color in this figure legend, the reader is referred to the web version of this article.)
A
Proportion of each category of metabolic pathways up-regulated in at least one epithelial cell subtype across tissues
Amino acid metabolism Carbohydrate metabolism Energy metabolism
Glycan biosynthesis and metabolism Lipid metabolism
Metabolism of cofactors and vitamins Metabolism of other amino acids Metabolism of terpenoids and polyketides Nucleotide metabolism
Xenobiotics biodegradation and metabolism
B
30
25
20
15
10
5
0
Fig. 4. Metabolic features of epithelial cells in the nine examined tissues of dairy cattle. (A) The pie chart shows the proportion of each category of metabolic pathways upregulated in the epithelial cell subtypes in the nine tissues. (B) Bar plots showing the numbers of each category of metabolic pathways upregulated in each epithelial cell subtype. BC: basal cell; cg-like SC: channel-gap-like spinous cell; GC: granule cell; SC: spinous cell.
the ileum and rectum, the Paneth cell types had the largest number of upregulated metabolic pathways (Fig. 4B). We also noted that pathways relating to ‘‘xenobiotics biodegradation and metabolism” and ‘‘glycan biosynthesis and metabolism” were predominantly enriched in the four-chambered stomach and intestinal cell sub- types, respectively. Our results suggest that each epithelial cell subtype could exert unique metabolic functions in cattle.
To further characterize metabolic heterogeneity at the single- cell level, we investigated metabolic fingerprints of epithelial cell subtypes across multiple tissues using a computational pipeline
[15] based on scRNA-seq data. Among the 60 identified metabolic pathways showing high activity (pathway activity score greater than 1 and permutation test p-value < 0.01) in at least one cell sub- type compared to other cell subtypes, only 5 covered more than half of the cell subtypes (Fig. 5A; Table S14), indicating that each cell subtype undergoes distinct metabolic reprogramming. For example, the cg-like SC subtypes had high activities of retinol metabolism in the rumen and reticulum. The arachidonic acid metabolism pathway, which is involved in maintaining mucosal integrity [26], was enriched in the pit cell subtypes and neck cell_2
in the abomasum (Fig. 5A). The goblet subtypes of the ileum and rectum both had high activities of mucin type O-glycan biosynthe- sis, which plays an important role in intestinal homeostasis. The starch and sucrose metabolism and mannose-type O-glycan biosynthesis pathways had the highest activities in the KLK1+ duct and ACHE+ acinar cell types of the salivary gland, respectively (Fig. 5A). In addition, we also found that some metabolic pathways with high activities were shared by multiple epithelial cell sub- types of the gastrointestinal tissues. For example, the ‘‘thiamine metabolism” pathway had high activities in almost all epithelial cell subtypes of forestomach tissues, which could also be sup- ported by high relative concentrations of the L-cysteine in the rumen, reticulum, and omasum epithelium (Fig. 5B) [27]. The epithelial cell subtypes of lower gut tissues had high activities of the ‘‘nicotinate and nicotinamide metabolism” pathway that could also be supported by high relative concentrations of the maleic acid (Fig. 5C), L-aspartic acid (Fig. 5D), and 4-aminobutyric acid (Fig. 5E) in these tissues [28]. Together, these results uncover the specific cellular metabolic features of each epithelial cell subtype
Metabolism of xenobiotics by cytochrome P450 Sulfur metabolism
Thiamine metabolism One carbon pool by folate
Drug metabolism - other enzymes Glycine, serine and threonine metabolism Porphyrin and chlorophyll metabolism Fatty acid biosynthesis
Propanoate metabolism Pyruvate metabolism
Steroid hormone biosynthesis
Ubiquinone and other terpenoid-quinone biosynthesis Valine, leucine and isoleucine degradation Tryptophan metabolism
Fatty acid degradation Retinol metabolism Tyrosine metabolism
Drug metabolism - cytochrome P450 Steroid biosynthesis
Biosynthesis of unsaturated fatty acids Glycosphingolipid biosynthesis - ganglio series Inositol phosphate metabolism
Sphingolipid metabolism
Amino sugar and nucleotide sugar metabolism N-Glycan biosynthesis
Glycosaminoglycan biosynthesis - heparan sulfate / heparin Other glycan degradation
Nicotinate and nicotinamide metabolism Glycosaminoglycan degradation Arginine biosynthesis
Mucin type O-glycan biosynthesis Arachidonic acid metabolism Starch and sucrose metabolism
Mannose type O-glycan biosynthesis Lysine degradation
Glycerolipid metabolism
Pentose and glucuronate interconversions Pentose phosphate pathway
Citrate cycle (TCA cycle) Glycolysis / Gluconeogenesis Oxidative phosphorylation Folate biosynthesis
Terpenoid backbone biosynthesis Pyrimidine metabolism
Purine metabolism Glutathione metabolism Fatty acid elongation Galactose metabolism
Glyoxylate and dicarboxylate metabolism beta-Alanine metabolism
Alanine, aspartate and glutamate metabolism Butanoate metabolism
Primary bile acid biosynthesis Fructose and mannose metabolism
Glycosylphosphatidylinositol (GPI)-anchor biosynthesis Arginine and proline metabolism
Cysteine and methionine metabolism Ether lipid metabolism Selenocompound metabolism Glycerophospholipid metabolism
Pathway activity 2.5
2
1.5
1
0.5
0
B
6.4
L-Cysteine
P = 0.066
P = 0.032
P = 0.012
C
7.7
7.5
P = 0.011
Maleic acid
P = 0.062
P = 0.0025
P = 0.0018
P = 0.0005
6.0
5.6
5.2
1
0
7.3
7.1
6.9
6.7
6.5
1
0
P = 0.053
P = 0.0004
P = 0.038
D
7.4
7.2
7.0
Rumen Reticulum Omasum Abomasum Ileum Rectum
L-Aspartic acid
P = 0.059
P = 0.029
P = 0.062
P = 0.030
E
7.0
6.5
6.0
Rumen Reticulum Omasum Abomasum Ileum Rectum
4-Aminobutyric acid
P = 0.021
P = 0.020
P = 0.0034
P = 0.044
6.8 5.5
6.6 5.0
6.4
1
0
Rumen Reticulum Omasum Abomasum Ileum
Rectum
4.5
1
0
Rumen Reticulum Omasum Abomasum Ileum
Rectum
Fig. 5. Cell type-specific metabolic features across tissues of dairy cattle. (A) Metabolic pathway activities of epithelial cell subtypes in the nine tissues. Statistically nonsignificant values (random permutation test P greater than 0.05) are shown as blanks. (B, C, D, E) The relative concentration of the L-Cysteine (B), Maleic acid (C), L- Aspartic acid (D), 4-Aminobutyric acid (E) in the epithelium of gastrointestinal tissues. The data in figure are presented as the means ± SD, and n = 3 for each tissue type. BC: basal cell; cg-like SC: channel-gap-like spinous cell; GC: granule cell; SC: spinous cell.
that enable them to survive and affect specific tissue microenvironments.
Cell-type-specific expression of solute carrier genes across tissues
Little is known about the roles of each cell type and their inter- play in the uptake of nutrients. As SCFAs are the major energy source for ruminants, we first analyzed the expression of solute carrier (SLC) genes that were related to SCFA absorption within hepatocytes and epithelial cell types in our dataset. SLC16A1, hav- ing a proven role in SCFA uptake [29], was highly expressed in specific cell types, including cg-like SC_1, cg-like SC_2, BC_2, and SC_2 in the rumen (Fig. 6A); MC, BC_1, and cg-like SC in the retic- ulum (Fig. 6B); MC, BC, and SC_1 in the omasum (Fig. 6C); pit cell_1 and pit cell_2 in the abomasum (Fig. 6D); and CCK+ enterocytes in the ileum and rectum (Fig. 6E and F). Other SLC16A family genes were also cell-type-specifically expressed, for example, SLC16A11 in the neck cell_2 of the abomasum (Fig. 6D), enteroendocrine cells of the ileum (Fig. 6E), goblet cells of the rectum (Fig. 6F), cholangio- cyte of the liver (Fig. 6G), basal and KLK1+ duct cell subtypes of the salivary gland (Fig. 6H), and luminal cell_1 of the mammary gland (Fig. 6I); SLC16A9 in the MC_1 of the rumen; and SLC16A7 in the LYSB+ and CLCA1+ goblet cell subtypes of the ileum.
A second and likely more important mechanism for SCFA absorption is the exchange of SCFAs for bicarbonate and other ions via antiporters, although the transporter has not been identified [30–31]. SLC genes encoding anion exchangers could be considered candidates for SCFA uptake transporters, including members of the SLC4A, SLC26A, SLC21A, and SLC22A families. As the disappearance of chloride from the mucosal side has been reported to correlate negatively with the uptake of SCFAs [30], we also focused on the SLC12A family, which is related to the transmembrane transport of chloride. The genes in these families were highly cell-type- specific. Interestingly, members of the SLC4A and SLC12 families were enriched in the cell subtypes with high expression of the SLC16A family genes mentioned above along the gastrointestinal tract (Fig. 6A-F). For instance, SLC4A9, encoding the most likely antiporter [32], and SLC12A7, predicted to be involved in fatty acid transmembrane transport [33], were both highly expressed in the cg-like SC_2 of the rumen and the cg-like SC of the reticulum, and SLC4A4 and SLC12A2 were both highly expressed in SC_1 of the omasum.
The SLC genes related to sugar transport, such as genes of the SLC2A and SLC45A families, were not enriched in almost all epithe- lial cell subtypes of forestomach tissues but were enriched in the specific cell subtypes of the other tissues in our study (Fig. 6A-F). This is consistent with the existing knowledge that forestomach epithelial cells take up only a small amount of glucose, amounting to<0.1% of the metabolizable energy intake [34]. Glucogenic pre- cursors, especially propionate, give rise to gluconeogenesis to meet the needs for hepatic glucose production in dairy cattle [35]. We found that hepatocytes uniquely highly expressed SLC2A3 (Fig. 6G), indicating that this gene plays a vital role in excreting glucose from the liver. We also noted that the SLC genes involved in amino acid transport were differentially expressed along the mammary gland epithelial cell subtypes. We identified the light chain amino acid transporter SLC3A2, the neutral amino acid trans- porter SLC38A2, and the cationic amino acid transporter SLC7A4 to be expressed in luminal cell_1, luminal cell_2, and luminal cell_3, respectively (Fig. 6I).
Moreover, corresponding to the gene expression, cell-type- enriched functions across different tissues were also observed. For instance, the GSEA showed that cg-like SC_2 of the rumen (Fig. 7A), the cg-like SC of the reticulum (Fig. 7B), and the SC_1 of the omasum (Fig. 7C) may have a high potential capacity for SCFA uptake. Gene set score analysis also identified the epithelial
cell subtypes that had high monocarboxylic acid transport scores along the gastrointestinal tract (Figs. S9 and S10). The saliva plays an important role in conducing to SCFA absorption [31], and the epithelial cell subtypes of salivary gland showed significantly dif- ferences in terms of saliva secretion (Fig. 7D). Collectively, we dis- covered cell subtypes with specific expression of SLC gene-encoded transporters that are related to the secretory and absorptive capac- ities of nutrients in each of the examined tissues in dairy cattle (Fig. 7E).
Relationship between Th17 cells and transport functions of forestomach epithelial cells
By analyzing the distribution of major immune cell types in var- ious tissues, we found that Th17 cells (highly expressing CD4 and IL17A) were rumen-, reticulum-, and omasum-specific cell types and accounted for the majority of total immune cells in these three tissues (Fig. 8A; Fig. S11A). Th17 cells were further confirmed by using fluorescence in situ hybridization (Fig. S11B and C). Depend- ing on their functional plasticity, Th17 cells are classified as home- ostatic Th17 cells or inflammatory pathogenic Th17 cells [36]. Homeostatic Th17 cells typically reside on mucosal surfaces to exert their effects [37], whereas inflammatory, pathogenic Th17 cells are required for the expression of IL23R [36]. Th17 cells rarely expressed IL23R or proinflammatory genes (Fig. S11D), indicating that they exist under homeostatic conditions in forestomach tis- sues. We next constructed intercellular communication networks using CellChat [16] (version 0.5.0) to uncover the cellular interac- tions in the rumen, reticulum, and omasum. Interestingly, Th17 cells predominantly interacted with the epithelial cell subtypes with high potential capacity for SCFA uptake discussed above in all three forestomach tissues through the IL-17 signaling pathway (Fig. 8B-D). Notably, among all the known ligand-receptor pairs, IL- 17 signaling in the rumen, reticulum, and omasum was attributed to IL17F and IL17A ligands and their multimeric IL17RA/IL17RC receptors (Fig. 8E-G).
Additionally, there is a distinct, tissue-specific pattern of IL-17 signaling-dependent genes that provides the basis for important physiologic functions [38]. To further investigate the cell response to IL-17 signaling, we separated epithelial cells with simultane- ously high expression levels of IL17RA and IL17RC (IL17RA and IL17RC expression levels both higher than 0.25) into the IL17Rhigh- IL17RChigh group and the other cells into the control group in each forestomach tissue (Fig. 9A-C). By comparison, we identified the upregulated DEGs in the IL17RAhighIL17RChigh group and then revealed Gene Ontology (GO) terms based on the upregulated DEGs. Surprisingly, we found that the upregulated DEGs of the IL17RAhighIL17RChigh group in the rumen, reticulum, and omasum were all enriched in GO terms associated with transport functions, especially the SCFAs in transmembrane transport (Fig. 9D-F). For example, monocarboxylic acid transport binding and transmem- brane transport were upregulated in the IL17RAhighIL17RChigh group in the forestomach tissues (Fig. 9D-F). These results indicate that Th17 cells play a vital role in regulating nutrient transport of IL17RAhighIL17RChigh epithelial cells in the rumen, reticulum, and omasum.
Discussion
Although notable progress has been made in describing the molecular features of bovine tissues [39], little is known about the diversity of cellular populations in complex tissues. Construct- ing high-dimensional molecular profiles across multiple cell types/subtypes by way of traditional molecular biological tech- niques such as bulk sequencing remains challenging. In the present
A
SLC2A1 SLC2A11 SLC26A6 SLC26A11 SLC12A2 SLC12A4 SLC12A7 SLC4A2 SLC4A7 SLC4A9 SLC16A9 SLC16A1
Rumen
Percent Expressed (%)
0
25
50
75
Average Expression
2.0
1.5
1.0
0.5
0.0
B
SLC45A2 SLC2A1 SLC2A3 SLC2A11 SLC12A4 SLC12A7 SLC12A9 SLC4A2 SLC4A7 SLC4A9 SLC16A9 SLC16A1
Reticulum
Percent Expressed (%)
0
25
50
75
100
Average Expression
2.0
1.5
1.0
0.5
0.0
C D Abomasum
SLC2A3 SLC2A1 SLC12A7 SLC12A4 SLC12A2 SLC26A11 SLC4A9 SLC4A7 SLC4A4 SLC4A2 SLC16A9 SLC16A1
Omasum
Percent Expressed (%)
0
25
50
75
Average Expression
2.0
1.5
1.0
0.5
0.0
SLC45A3 SLC2A1 SLC12A7 SLC12A2 SLC4A7 SLC4A4 SLC4A2 SLC16A11 SLC16A1
Percent Expressed (%)
10
20
30
40
50
Average Expression
1.5
1.0
0.5
0.0
E
SLC51B SLC51A
Ileum
Percent Expressed (%)
F
SLC51B
SLC51A
Rectum
SLC27A5 SLC2A8 SLC2A3 SLC12A8 SLC12A7 SLC12A2 SLC26A11 SLC26A7 SLC26A3 SLC4A4 SLC4A7 SLC4A2 SLC16A12 SLC16A11 SLC16A7 SLC16A1
0
25
50
75
100
Average Expression
2.0
1.5
1.0
0.5
0.0
SLC27A5 SLC2A8 SLC2A3 SLC12A8 SLC12A7 SLC12A2 SLC26A11 SLC26A7 SLC26A3 SLC4A4 SLC4A7 SLC4A2 SLC16A12 SLC16A11 SLC16A7 SLC16A1
Percent Expressed (%)
0
25
50
75
Average Expression
2.0
1.5
1.0
0.5
0.0
G
SLC5A12
Liver
Percent Expressed (%)
H
SLC5A8
Salivary gland
Percent
I
SLC27A6 SLC27A5 SLC27A1
Mammary gland
Percent Expressed (%)
SLC5A1
SLC2A3 SLC2A2 SLC2A1 SLC12A7 SLC12A2 SLC4A7 SLC4A4 SLC4A2 SLC16A12 SLC16A11 SLC16A7 SLC16A1
0
20
40
60
80
Average Expression
0.6
0.4
0.2
0.0
SLC2A8
SLC2A13 SLC2A11 SLC2A4 SLC2A1 SLC12A9 SLC26A6 SLC4A4 SLC4A7 SLC4A2 SLC16A11 SLC16A3 SLC16A1
Expressed (%)
0
25
50
75
Average Expression
1.5
1.0
0.5
0.0
SLC7A8
SLC7A4 SLC38A2 SLC38A1 SLC43A1 SLC1A5 SLC1A1 SLC3A2 SLC6A14 SLC2A8 SLC2A3 SLC2A1 SLC12A7 SLC12A2 SLC4A7 SLC4A2 SLC16A11 SLC16A1
25
50
75
Average Expression
0.9
0.6
0.3
0.0
Fig. 6. Cell-type-enriched expression of solute carrier (SLC) genes across different tissues. (A-I) Dot plots showing the gene expression of solute carriers in the rumen (A), reticulum (B), omasum (C), abomasum (D), ileum (E), rectum (F), liver (G), salivary gland (H), and mammary gland (I) datasets. BC: basal cell; cg-like SC: channel-gap-like spinous cell; GC: granule cell; MC: mitotic cell; SC: spinous cell; TA: transit-amplifying cell.
A
0.8
0.6
Monocarboxylic acid transport
p.adjust = 0.0034
B
0.8
0.6
Monocarboxylic acid transport
p.adjust = 0.0037
0.4 0.4
0.2 0.2
0.0 0.0
2
1
0
−1
5000 10000 15000
cg-like SC_2
CMonocarboxylic acid transport
2
1
0
−1
5000 10000 15000
cg-like SC
DSaliva secretion
0.8
0.6
0.4
0.2
0.0
2
1
0
−1
p.adjust = 0.0454
5000 10000 15000
SC_1
0.6 c a b
0.4
0.2 d
0
-0.2 e
-0.4
E
Rumen
Gastrointestinal tract
Microorganism
SCFAs
Ileum Rectum
Reticulum Omasum Abomasum Small intestine Large intestine
cg-like SC_1 cg-like SC_2 cg-like SC
Mammary gland
SC_1
Pit cell_2
CCK+ enterocyte
Milk
SCFAs
HCO3-
Glucose
Amino acids
SCFAs
Luminal cells
Gluconeogenesis
Liver
Hepatocyte
SLC16A1 SLC12A7 SLC4A9 SLC12A2 SLC4A4 SLC26A3 SLC4A7
SLC2A3 SLC16A11
SLC3A2 SLC38A2 SLC2A1 SLC7A4
SLC27A6
Fig. 7. Cell-type-enriched functions across different tissues. (A-C) GSEA identified the biological processes enriched in cg-like SC_2, cg-like SC, and SC_1 in the rumen (A), reticulum (B), and omasum (C), respectively. (D) Gene scoring analysis for epithelial cell subtypes of salivary gland using the ‘‘Saliva secretion” gene set. Mean values labeled without a common letter were defined as significantly different (the order of the letters was sorted according to mean value from high to low, adjusted p value < 0.05). (E) The roles of representative cell subtypes with specific expression of SLC gene-encoded transporters across multiple tissues. cg-like SC: channel-gap-like spinous cell; SC: spinous cell.
study, we performed single-cell transcriptomic analysis to identify the high-resolution landscapes of the 10 key metabolic tissue types in dairy cattle. As the rumen, reticulum, and omasum are unique
organs in cattle and other ruminants, to our knowledge, there is no existing protocol to dissociate forestomach tissues into single- cell suspensions for scRNA-seq. In this study, we developed cell
Rumen Reticulum Omasum Abomasum
Ileum Rectum Liver
Salivary gland Mammary gland
Blood
Percent (%)
25
50
75
B IL-17 signaling in rumen
SC_3
BC_1
γδ T
cg-like SC_1
SC_2 SC_1
GC_4
GC_3
GC_2
E
IL17F − (IL17RA+IL17RC)
Contribution of each L−R pair
GC_5
MC_1 BC_2
cg-like SC_2
MC_2
C
GC_1
Th17 BVEC
cDC2 MKI67+ Th17
cg-like SC_3 GC_6 SC_4 CD4- CD8- T
IL17AF − (IL17RA+IL17RC) IL17A − (IL17RA+IL17RC)
Relative contribution
IL-17 signaling in reticulum F
cg-like SC GC_3
BC_2
GC_2
γδ T
BC_1
SC_1
IL17F − (IL17RA+IL17RC)
Contribution of each L−R pair
MC BC_3
cDC2
SC_2
Th17
GC_1 VSMC
CD4- CD8- T MKI67+ Th17
IL17AF − (IL17RA+IL17RC) IL17A − (IL17RA+IL17RC)
Relative contribution
D IL-17 signaling in omasum
G
IL17F − (IL17RA+IL17RC) IL17AF − (IL17RA+IL17RC) IL17A − (IL17RA+IL17RC)
Contribution of each L−R pair
Relative contribution
Fig. 8. Cell-to-cell communications of Th17 cells and epithelial cells in the forestomach tissues. (A) Dot plots showing the proportions of major immune cell types across multiple tissues. (B-D) The inferred IL-17 signaling networks in the rumen (B), reticulum (C), and omasum (D) datasets. Circle sizes are proportional to the number of cells in each cell group. Edge width represents the communication probability. (E-G) Relative contribution of each ligand-receptor pair to the overall communication network of the IL-17 signaling pathway in the rumen (E), reticulum (F), and omasum (G) datasets. BC: basal cell; cg-like SC: channel-gap-like spinous cell; BVEC: blood vascular endothelial cell; cDC1: conventional type 1 dendritic cell; cDC2: conventional type 2 dendritic cell; GC: granule cell; DC: dendritic cell; LEC: lymphatic endothelial cell; MC: mitotic cell; NK: natural killer cell; NKT: natural killer T cell; SC: spinous cell; Tfh: T follicular cell; VSMC: vascular smooth muscle cell.
A1527 IL17RAhigh IL17RChigh rumen epithelial cells
2.0
1.5
1.0
D
Rumen IL17RAhigh IL17RChigh epithelial cells upregulated GO terms
0.5
0.0
0.0 0.5 1.0 1.5 2.0 2.5
0 1 2 3
IL17RA expression
B548 IL17RAhigh IL17RChigh reticulum epithelial cells
-log10(p value)
E
Reticulum IL17RAhigh IL17RChigh epithelial cells upregulated GO terms
2.0
1.5
1.0
0.5
0.0
0 1 2
0 2 4 6
-log10(p value)
C
2.0
IL17RA expression
448 IL17RAhigh IL17RChigh omasum epithelial cells
F
Omasum IL17RAhigh IL17RChigh epithelial cells upregulated GO terms
1.5
1.0
0.5
0.0
0.0
0.5
1.0
1.5
2.0
2.5
0 1 2 3
IL17RA expression
-log10(p value)
Fig. 9. Th17 cell-epithelial interactions mediate the forestomach response to nutrient transport in dairy cattle. (A-C) Expression levels of the IL17RA and IL17RC receptors used for separating epithelial cells into the IL17RAhighIL17RChigh group and the control group in the rumen (A), reticulum (B), and omasum (C) datasets. (D-F) Representative upregulated GO terms in the IL17RAhighIL17RChigh cells in the rumen (D), reticulum (E), and omasum (F).
isolation approaches for these tissues (see Material and methods and Fig. S1B and C) and obtained high-quality cell suspensions. Cell type definition is another major challenge in scRNA-seq studies of bovine metabolic tissues. We carefully studied and referenced pre- vious studies [19–22,40–67] to generate a list of canonical cell- type-specific markers for each cell type with manual curation
(Table S1). Moreover, due to the limited availability of markers with specificity to bovine cell lineages, many of the markers in the list of canonical cell-type-specific markers were drawn from murine and human datasets. Therefore, to further assess whether the marker genes were sufficient to serve as a classifier for cell identity, the AUC values of highly expressed marker genes were
calculated and assisted in cell type annotation (see Material and methods). We created integrated pipelines of scRNA-seq in cattle metabolic tissues, especially for uncharacterized forestomach tis- sues. The results provide a comprehensive view of cellular classifi- cation and fill the gap regarding the limited availability of cell- specific markers in bovine tissues. The data in this study also offer a comparative framework for future studies using models of cattle or other ruminant animals.
The death and renewal of cells occurs continuously not only in the columnar epithelium of the abomasum, small intestine, and large intestine but also in the stratified squamous epithelium of the forestomach tissues, and lifelong self-renewal in these epithe- lial cells relies on the activity of stem cells/progenitors [24,68]. Here, we discovered MC, the epithelial progenitor-like subtypes present in the rumen, reticulum, and omasum. The forestomach epidermal proliferation and differentiation events are believed to coordinate with SCFA absorption, and the formation of the cor- neum acts as a protective barrier [24,69]. We found that MC, BC, SC, and GC subtype-specific highly expressed transcription factors (Fig. S6) could clearly distinguish these epithelial cell subtypes in each forestomach tissue, indicating that these transcription factors represent a certain extent of population variance. As few studies have reported the exact mechanisms controlling how forestomach epithelial cells differentiate, whether these transcription factors are involved in this process needs to be further elucidated and experimentally validated.
Cellular metabolism in epithelial cells supports specific demands for energy metabolism and functional maintenance across key metabolic tissues to optimize milk production during lactation. However, if deficient in nutrient absorption, metabolic disorders will occur in lactating dairy cattle that have very high nutritional requirements. Research in recent decades has provided insights into a broad set of diverse nutrient absorptive functions in dairy cattle, but the rumen has been the focal point of research, and other key metabolic tissues have received less attention [31,69]. Although direct observations of cell metabolism in vivo are difficult at single-cell resolution, it is commonly accepted that scRNA-seq, an indirect means of assessing metabolism, could provide impor- tant insights into metabolism at the single-cell level [15]. In the current study, we created a global picture of the metabolic features of several epithelial cell subtypes along the gastrointestinal tract as well as other key metabolic tissues (liver, salivary gland, and mam- mary gland) by analyzing single-cell datasets. Metabolic plasticity is tissue-specific, and the activity of dynamic metabolic pathways with high plasticity is largely dependent on specific cell subtypes in the different tissues. We focused on SLC membrane transport proteins because they are the largest group of transporters that import and export most nutrients, such as sugars, SCFAs, and amino acids [70]. SLCs are ubiquitously distributed throughout tis- sues, and those associated with metabolic homeostasis are indis- pensable for supporting lactation [71]. Instead of glucose, the SCFAs released as the major end products of microbial fermenta- tion in the reticulorumen are absorbed through the epithelium as a major energy source in dairy cattle [31,35]. Although previous studies in recent decades have provided further insight into differ- ent uptake pathways for SCFAs in cattle [31–32], the SCFA trans- porters and their cellular distribution have not been clearly identified. We found that the mean expression of SLC genes encod- ing candidates for SCFA transmembrane transport was cell-type- specific in forestomach tissues as well as other tissues in our study in which the cell subtypes with high levels of these genes in forestomach tissues were mainly basale and spinosum strata cells, consistent with previous studies [72–73]. We noted that co- expression patterns of the SLC genes in epithelial cells across mul- tiple tissues in the present study, such as SLC16A1, SLC4A9, and SLC12A7, were highly expressed simultaneously in the cg-like SC2
of the rumen and the cg-like SC of the reticulum. In different large-scale datasets, the SLC co-expression patterns also have high robustness [70]. SLC function may have a high extent of interde- pendence, reflecting the integrative nature of metabolism required for homeostatic stability, but experimental validation of this hypothesis will be required. In short, our scRNA-seq data fill this gap and provide an opportunity for investigation of the cell-type- specific roles in nutrient absorption in each tissue at single-cell resolution. The cell subtypes that have preferential absorption of different nutrients and their highly expressed marker genes that could be considered in selecting high milk production dairy cows. Moreover, single-cell RNA-seq data can be integrated with other omics datasets, such as ChIP-seq or SNPs, to build transcriptional regulatory networks at cell-type resolution and further identify candidate loci associated with milk yield traits. These candidate molecular markers will assist in breeding programs, or be geneti- cally modified to test their effects on the milking traits.
The key metabolic tissues, including the gastrointestinal tract, liver, salivary gland, and mammary gland tissues, of dairy cattle that were examined in our study might balance nutrient transport with defending the barrier. A recent study reported that immune cells play an important role in regulating the intestinal response to nutrient sensing [74]. Therefore, we aimed to explore the inter- actions between cell subtypes within particular tissues. During our comprehensive analysis, we identified a role for Th17 cells in reg- ulating nutrient transport of epithelial cells in the rumen, reticu- lum, and omasum. IL17F and IL17A, produced by Th17 cells, play an important role in the regulation of gene expression in SCFA transport specialized epithelial cells in the forestomach. The forestomach epithelial cells in response to IL17A and IL17F highly expressed IL17RA and IL17RC simultaneously. Despite being exclu- sively in silico, the predictions remain biologically significant by restricting analysis to the only highly expressed receptor-ligand interactions. Indeed, IL-17RA partners with IL-17RC to induce a response to IL-17F and IL-17A [75]. Although the focus of previous studies on IL-17 signaling was limited to the activation of down- stream pathways to induce the expression of antimicrobial pep- tides in epithelial cells of cattle [76–77], recent studies have shown evidence of a tissue-specific pattern of IL-17-dependent genes that underlies the diversity of physiologic functions of this cytokine [38]. For instance, IL-17 signaling promotes intestinal epithelial regeneration and barrier integrity, while inhibition of IL-17 receptors is associated with decreased epithelial proliferation and increased epithelial permeability [78]. An important role of Th17 cells in regulating the nutrient transport of IL17RAhigh- IL17RChigh epithelial cells of forestomach tissues represents another example of a distinct, tissue-specific pattern of IL-17- dependent genes. Nevertheless, the mechanisms underlying how ruminal, reticular, and omasal epithelial cells respond to IL17F and IL17A secreted by Th17 cells remain largely unexplored.
In addition to these notable findings, our study is not without limitations. First, the dissociation bias inherent in all single-cell tis- sue experiments may cause spurious changes in cell distribution and limit our ability to compare the proportions of different cell types in a tissue. As dissociation does not equally affect all cell types in a tissue, changes in the relative composition of a given cell type across tissues might be more meaningful than comparing pro- portions of different cell types in a single tissue. Second, although comprehensive cellular classification in multiple tissues was pro- vided in our study and verified the presence of some key cell sub- types, unfortunately, the limited availability of useful immunofluorescence reagents such as monoclonal or polyclonal antibodies with specificity to bovine cells has hindered the exper- imental validation of other cell types. RNA probes specific to bovine cells will be considered for verification experiments. Third, the current analysis is limited in sequencing depth, which is a lim-
itation intrinsic to the 10X Genomics system. However, we believe that this issue will be improved with the advancement of sequenc- ing technology in the future.
Conclusion
In summary, we built a single-cell transcriptomic atlas of key metabolic tissues in lactating dairy cattle, which provides a valu- able resource for uncovering distinct gene expression patterns of different tissues at single-cell resolution. Importantly, the identi- fied intercellular communication signaling pathways are critical for nutrient transport. These findings will help to reveal the key factors and develop potential precise targets for the improvement of animal health and production.
CRediT authorship contribution statement
Jia-Jin Wu: Conceptualization, Methodology, Validation, Formal analysis, Investigation, Writing – original draft, Visualization. Sen- lin Zhu: Methodology, Software, Formal analysis, Investigation, Visualization. Fengfei Gu: Validation, Investigation, Visualization. Teresa G. Valencak: Resources, Supervision. Jian-Xin Liu: Concep- tualization, Resources, Supervision. Hui-Zeng Sun: Conceptualiza- tion, Methodology, Formal analysis, Investigation, Supervision, Writing – original draft, Writing – review & editing.
Declaration of Competing Interest
The authors declare that they have no known competing finan- cial interests or personal relationships that could have appeared to influence the work reported in this paper.
Acknowledgments
We thank LC Sciences and Wuhan Institute of Biotechnology for deep sequencing experiments. The authors would also like to thank the Core Facilities, Zhejiang University School of Medicine, for technical support and our colleagues Ming-Yuan Xue, Yi-Fan Zhong, and Lu-Yi Jiang at the Institute of Dairy Science, College of Animal Sciences of Zhejiang University, for their assistance with sampling. This research was supported by the National Natural Science Foundation of China (32002207 and 31729004, Beijing), the Fundamental Research Funds for the Zhejiang Provincial Universities (2021XZZX027), the China Agriculture (Dairy) Research System (CARS-36, Beijing), and the ‘‘Hundred Talents Pro- gram” Research Professor Start-up Fund of Zhejiang University.
Appendix A. Supplementary material
Supplementary data to this article can be found online at https://doi.org/10.1016/j.jare.2021.11.009.
References
[1]Kearney J. Food consumption trends and drivers. Philos Trans R Soc Lond B Biol Sci 2010;365(1554):2793–807.
[2]Ortiz-Bobea A, Ault TR, Carrillo CM, Chambers RG, Lobell DB. Anthropogenic climate change has slowed global agricultural productivity growth. Nat Clim Change 2021;11(4):306–12.
[3]Connor EE, Li RW, Baldwin RL, Li C. Gene expression in the digestive tissues of ruminants and their relationships with feeding and digestive processes. Animal 2010;4(7):993–1007.
[4]Flint HJ, Bayer EA. Plant cell wall breakdown by anaerobic microorganisms from the mammalian digestive tract. Ann N Y Acad Sci 2008;1125:280–8.
[5]Allen MS. Relationship Between Fermentation Acid Production in the Rumen and the Requirement for Physically Effective Fiber. J Dairy Sci 1997;80 (7):1447–62.
[6]
Sun HZ, Zhou M, Wang O, Chen Y, Liu JX, Guan LL. Multi-omics reveals functional genomic and metabolic mechanisms of milk production and quality in dairy cows. Bioinformatics 2020; 36:2530-2537.
[7]Ding J, Adiconis X, Simmons SK, Kowalczyk MS, Hession CC, Marjanovic ND, et al. Systematic comparison of single-cell and single-nucleus RNA-sequencing methods. Nat Biotechnol 2020;38(6):737–46.
[8]Xue R, Li R, Bai F. Single cell sequencing: technique, application, and future development. Sci Bull 2015;60(1):33–42.
[9]Macosko E, Basu A, Satija R, Nemesh J, Shekhar K, Goldman M, et al. Highly parallel genome-wide expression profiling of individual cells using nanoliter droplets. Cell 2015;161(5):1202–14.
[10]Hao Y, Hao S, Andersen-Nissen E, Mauck WM, Zheng S, Butler A, et al. Integrated analysis of multimodal single-cell data. Cell 2021;184 (13):3573–3587.e29.
[11]McGinnis CS, Murrow LM, Gartner ZJ. DoubletFinder: Doublet Detection in Single-Cell RNA Sequencing Data Using Artificial Nearest Neighbors. Cell Syst 2019;8(4):329–337.e4.
[12]Korsunsky I, Millard N, Fan J, Slowikowski K, Zhang F, Wei K, et al. Fast, sensitive and accurate integration of single-cell data with Harmony. Nat Methods 2019;16(12):1289–96.
[13]Yu G, Wang L-G, Han Y, He Q-Y. clusterProfiler: an R package for comparing biological themes among gene clusters. Omics 2012;16(5):284–7.
[14]Cao J, Spielmann M, Qiu X, Huang X, Ibrahim DM, Hill AJ, et al. The single-cell transcriptional landscape of mammalian organogenesis. Nature 2019;566 (7745):496–502.
[15]Xiao Z, Dai Z, Locasale JW. Metabolic landscape of the tumor microenvironment at single cell resolution. Nat Commun 2019;10:3763.
[16]Jin S, Guerrero-Juarez CF, Zhang L, Chang I, Ramos R, Kuan C-H, et al. Inference and analysis of cell-cell communication using Cell Chat. Nat Commun 2021;12
(1).doi: https://doi.org/10.1038/s41467-021-21246-9.
[17]He S, Wang L-H, Liu Y, Li Y-Q, Chen H-T, Xu J-H, et al. Single-cell transcriptome profiling of an adult human cell atlas of 15 major organs. Genome Biol 2020;21
(1).doi: https://doi.org/10.1186/s13059-020-02210-0.
[18]Chen L, Qiu Q, Jiang Yu, Wang K, Lin Z, Li Z, et al. Large-scale ruminant genome sequencing provides insights into their evolution and distinct traits. Science 2019;364(6446). doi: https://doi.org/10.1126/science:aav6202.
[19]Bond JJ, Donaldson AJ, Coumans JVF, Austin K, Ebert D, Wheeler D, et al. Protein profiles of enzymatically isolated rumen epithelium in sheep fed a fibrous diet. J Anim Sci Biotechnol 2019;10(1). doi: https://doi.org/10.1186/s40104-019- 0314-0.
[20]He H, Suryawanshi H, Morozov P, Gay-Mimbrera J, Del Duca E, Kim HJ, et al. Single-cell transcriptome analysis of human skin identifies novel fibroblast subpopulation and enrichment of immune subsets in atopic dermatitis. J Allergy Clin Immunol 2020;145(6):1615–28.
[21]Zou Z, Long X, Zhao Q, Zheng Y, Song M, Ma S, et al. A single-cell transcriptomic atlas of human skin aging. Dev Cell 2021;56(3):383–397.e8.
[22]Robitaille H, Proulx R, Robitaille K, Blouin R, Germain L. The mitogen-activated protein kinase kinase kinase dual leucine zipper-bearing kinase (DLK) acts as a key regulator of keratinocyte terminal differentiation. J Biol Chem 2005;280 (13):12732–41.
[23]Goodenough DA, Paul DL. Gap junctions. Cold Spring Harb Perspect Biol 2009;1(1).
[24]Yohe TT, Tucker HLM, Parsons CLM, Geiger AJ, Akers RM, Daniels KM. Short communication: Initial evidence supporting existence of potential rumen epidermal stem and progenitor cells. J Dairy Sci 2016;99(9):7654–60.
[25]Ballesteros I, Rubio-Ponce A, Genua M, Lusito E, Kwok I, Fernández-Calvo G, et al. Co-option of Neutrophil Fates by Tissue Environments. Cell 2020;183 (5):1282–1297.e18.
[26]Tallima H, El Ridi R. Arachidonic acid: Physiological roles and potential health benefits – A review. J Adv Res 2018;11:33–41.
[27]Jurgenson CT, Begley TP, Ealick SE. The structural and biochemical foundations of thiamin biosynthesis. Annu Rev Biochem 2009;78(1):569–603.
[28]Kanehisa M, Sato Y, Kawashima M. KEGG mapping tools for uncovering hidden features in biological data. Protein Sci 2021.
[29]Wang N, Jiang X, Zhang S, Zhu A, Yuan Y, Xu H, et al. Structural basis of human monocarboxylate transporter 1 inhibition by anti-cancer drug candidates. Cell 2021;184(2):370–383.e13.
[30]Aschenbach JR, Bilk S, Tadesse G, Stumpff F, Gäbel G. Bicarbonate-dependent and bicarbonate-independent mechanisms contribute to nondiffusive uptake of acetate in the ruminal epithelium of sheep. Am J Physiol Gastrointest Liver Physiol 2009;296(5):G1098–107.
[31]Baaske L, Gäbel G, Dengler F. Ruminal epithelium: A checkpoint for cattle health. J Dairy Res 2020;87(3):322–9.
[32]Xiang R, Oddy VH, Archibald AL, Vercoe PE, Dalrymple BP. Epithelial, metabolic and innate immunity transcriptomic signatures differentiating the rumen from other sheep and mammalian gastrointestinal tract tissues. PeerJ 2016; 4: e1762.
[33]Lachmann A, Torre D, Keenan AB, Jagodnik KM, Lee HJ, Wang L, et al. Massive mining of publicly available RNA-seq data from human and mouse. Nat Commun 2018;9(1). doi: https://doi.org/10.1038/s41467-018-03751-6.
[34]Gäbel G, Aschenbach JR, Müller F. Transfer of energy substrates across the ruminal epithelium: implications and limitations. Anim Health Res Rev 2002;3 (1):15–30.
[35]Aschenbach JR, Kristensen NB, Donkin SS, Hammon HM, Penner GB. Gluconeogenesis in dairy cows: the secret of making sweet milk from sour dough. IUBMB Life 2010;62(12):869–77.
[36]Wu L, Hollinshead KER, Hao Y, Au C, Kroehling L, Ng C, et al. Niche-selective inhibition of pathogenic Th17 cells by targeting metabolic redundancy. Cell 2020;182(3):641–654.e20.
[37]Kumar P, Monin L, Castillo P, Elsegeiny W, Horne W, Eddens T, et al. Intestinal interleukin-17 receptor signaling mediates reciprocal control of the gut microbiota and autoimmune inflammation. Immunity 2016;44(3):659–71.
[38]Amatya N, Garg AV, Gaffen SL. IL-17 Signaling: The Yin and the Yang. Trends Immunol 2017;38(5):310–22.
[39]Fang L, Cai W, Liu S, Canela-Xandri O, Gao Y, Jiang J, et al. Comprehensive analyses of 723 transcriptomes enhance genetic and biological interpretations for complex traits in cattle. Genome Res 2020;30(5):790–801.
[40]Amatschek S, Kriehuber E, Bauer W, Reininger B, Meraner P, Wolpl A, et al. Blood and lymphatic endothelial cell-specific differentiation programs are stringently controlled by the tissue environment. Blood 2007; 109:4777-4785.
[41]Storset A, Slettedal I, Williams J, Law A, Dissen E. Natural killer cell receptors in cattle: a bovine killer cell immunoglobulin-like receptor multigene family contains members with divergent signaling motifs. Eur J Immunol 2003;33 (4):980–90.
[42]Bach K, Pensa S, Grzelak M, Hadfield J, Adams DJ, Marioni JC, et al. Differentiation dynamics of mammary epithelial cells revealed by single-cell RNA sequencing. Nat Commun 2017;8:2128.
[43]Busslinger GA, Weusten BLA, Bogte A, Begthel H, Brosens LAA, Clevers H. Human gastrointestinal epithelia of the esophagus, stomach, and duodenum resolved at single-cell resolution. Cell Rep 2021;34(10):108819. doi: https:// doi.org/10.1016/j.celrep.2021.108819.
[44]Chen Y-P, Yin J-H, Li W-F, Li H-J, Chen D-P, Zhang C-J, et al. Single-cell transcriptomics reveals regulators underlying immune cell diversity and immune subtypes associated with prognosis in nasopharyngeal carcinoma. Cell Res 2020;30(11):1024–42.
[45]Duvel A, Frank C, Schnapper A, Schuberth HJ, Sipka A. Classically or alternatively activated bovine monocyte-derived macrophages in vitro do not resemble CD163/Calprotectin biased macrophage populations in the teat. Innate Immun 2012;18:886–96.
[46]Dwyer DF, Barrett NA, Austen KF. Immunological Genome Project C. Expression profiling of constitutive mast cells reveals a unique identity within the immune system. Nat Immunol 2016;17(7):878–87.
[47]Frie MC, Sporer KRB, Benitez OJ, Wallace JC, Droscha CJ, Bartlett PC, et al. Dairy Cows Naturally Infected with Bovine Leukemia Virus Exhibit Abnormal B- and T-Cell Phenotypes after Primary and Secondary Exposures to Keyhole Limpet Hemocyanin. Front Vet Sci 2017;4:112.
[48]Hamilton CA, Mahan S, Bell CR, Villarreal-Ramos B, Charleston B, Entrican G, et al. Frequency and phenotype of natural killer cells and natural killer cell subsets in bovine lymphoid compartments and blood. Immunology 2017;151 (1):89–97.
[49]Hamilton CA, Young R, Jayaraman S, Sehgal A, Paxton E, Thomson S, et al. Development of in vitro enteroids derived from bovine small intestinal crypts. Vet Res 2018;49(1). doi: https://doi.org/10.1186/s13567-018-0547-5.
[50]Han X, Chen H, Huang D, Chen H, Fei L, Cheng C, et al. Mapping human pluripotent stem cell differentiation pathways using high throughput single- cell RNA-sequencing. Genome Biol 2018;19(1). doi: https://doi.org/10.1186/ s13059-018-1426-0.
[51]Han X, Wang R, Zhou Y, Fei L, Sun H, Lai S, et al. Mapping the Mouse Cell Atlas by Microwell-Seq. Cell 2018;172(5):1091–1107.e17.
[52]Han X, Zhou Z, Fei L, Sun H, Wang R, Chen Y, et al. Construction of a human cell landscape at single-cell level. Nature 2020;581(7808):303–9.
[53]Hauser BR, Aure MH, Kelly MC, Genomics, Computational Biology C, Hoffman MP, et al. Generation of a Single-Cell RNAseq Atlas of Murine Salivary Gland Development. iScience 2020; 23:101838.
[54]Horst D, Gu X, Bhasin M, Yang Q, Verzi M, Lin D, et al. Requirement of the epithelium-specific Ets transcription factor Spdef for mucous gland cell function in the gastric antrum. J Biol Chem 2010;285(45):35047–55.
[55]Huang B, Chen Z, Geng L, Wang J, Liang H, Cao Y, et al. Mucosal Profiling of Pediatric-Onset Colitis and IBD Reveals Common Pathogenics and Therapeutic Pathways. Cell 2019;179(5):1160–1176.e24.
[56]Hussen J, Schuberth HJ. Heterogeneity of Bovine Peripheral Blood Monocytes. Front Immunol 2017;8:1875.
[57]
Liu Z, Jin YQ, Chen L, Wang Y, Yang X, Cheng J, et al. Specific marker expression and cell state of Schwann cells during culture in vitro. PLoS One 2015; 10: e0123278.
[58]Ma S, Sun S, Geng L, Song M, Wang W, Ye Y, et al. Caloric Restriction Reprograms the Single-Cell Transcriptional Landscape of Rattus Norvegicus Aging. Cell 2020;180(5):984–1001.e22.
[59]MacParland SA, Liu JC, Ma X-Z, Innes BT, Bartczak AM, Gage BK, et al. Single cell RNA sequencing of human liver reveals distinct intrahepatic macrophage populations. Nat Commun 2018;9(1). doi: https://doi.org/10.1038/s41467- 018-06318-7.
[60]Pizzolato G, Kaminski H, Tosolini M, Franchini DM, Pont F, Martins F, et al. Single-cell RNA sequencing unveils the shared and the distinct cytotoxic hallmarks of human TCRVdelta1 and TCRVdelta2 gammadelta T lymphocytes. Proc Natl Acad Sci U S A 2019;116:11906–15.
[61]Rabiger FV, Rothe K, von Buttlar H, Bismarck D, Buttner M, Moore PF, et al. Distinct Features of Canine Non-conventional CD4(-)CD8alpha(-) Double- Negative TCRalphabeta(+) vs. TCRgammadelta(+) T Cells. Front Immunol 2019;10(2748).
[62]Schelker M, Feau S, Du J, Ranu N, Klipp E, MacBeath G, et al. Estimation of immune cell content in tumour tissue using single-cell RNA-seq data. Nat Commun 2017;8(1). doi: https://doi.org/10.1038/s41467-017-02289-3.
[63]Schmid D, Zeis T, Sobrio M, Schaeren-Wiemers N. MAL overexpression leads to disturbed expression of genes that influence cytoskeletal organization and differentiation of Schwann cells. ASN Neuro 2014;6(5). doi: https://doi.org/ 10.1177/1759091414548916.
[64]Talker SC, Baumann A, Barut GT, Keller I, Bruggmann R, Summerfield A. Precise Delineation and Transcriptional Characterization of Bovine Blood Dendritic- Cell and Monocyte Subsets. Front Immunol 2018; 9:2505.
[65]Villani A-C, Satija R, Reynolds G, Sarkizova S, Shekhar K, Fletcher J, et al. Single- cell RNA-seq reveals new types of human blood dendritic cells, monocytes, and progenitors. Science 2017;356(6335). doi: https://doi.org/ 10.1126/science:aah4573.
[66]Wang Y, Song W, Wang J, Wang T, Xiong X, Qi Z, et al. Single-cell transcriptome analysis reveals differential nutrient absorption functions in human intestine. J Exp Med 2020; 217: e20191130.
[67]Young MD, Mitchell TJ, Vieira Braga FA, Tran MGB, Stewart BJ, Ferdinand JR, et al. Single-cell transcriptomes from human kidneys reveal the cellular identity of renal tumors. Science 2018;361(6402):594–9.
[68]Kim TH, Shivdasani RA. Stomach development, stem cells and disease. Development 2016;143:554–65.
[69]Steele MA, Penner GB, Chaucheyras-Durand F, Guan LL. Development and physiology of the rumen and the lower gut: Targets for improving gut health. J Dairy Sci 2016;99(6):4955–66.
[70]César-Razquin A, Snijder B, Frappier-Brinton T, Isserlin R, Gyimesi G, Bai X, et al. A Call for Systematic Research on Solute Carriers. Cell 2015;162 (3):478–87.
[71]Patel OV, Casey T, Plaut K. Profiling solute-carrier transporters in key metabolic tissues during the postpartum evolution of mammary epithelial cells from nonsecretory to secretory. Physiol Genomics 2019;51(11):539–52.
[72]Kent-Dennis C, Penner GB. Effects of a proinflammatory response on metabolic function of cultured, primary ruminal epithelial cells. J Dairy Sci 2021;104 (1):1002–17.
[73]Stumpff F. A look at the smelly side of physiology: transport of short chain fatty acids. Pflügers Arch 2018;470(4):571–98.
[74]Sullivan ZA, Khoury-Hanold W, Lim J, Smillie C, Biton M, Reis BS, et al. gammadelta T cells regulate the intestinal response to nutrient sensing. Science 2021;371(eaba8310).
[75]Gaffen SL. Structure and signalling in the IL-17 receptor family. Nat Rev Immunol 2009;9(8):556–67.
[76]Chase C, Kaushik RS. Mucosal Immune System of Cattle: All Immune Responses Begin Here. Vet Clin North Am Food Anim Pract 2019;35 (3):431–51.
[77]Pan X, Cai Y, Li Z, Chen X, Heller R, Wang N, et al. Modes of genetic adaptations underlying functional innovations in the rumen. Sci China Life Sci 2021;64 (1):1–21.
[78]Andrews C, McLean MH, Durum SK. Cytokine Tuning of Intestinal Epithelial Function. Front Immunol 2018;9:1270.